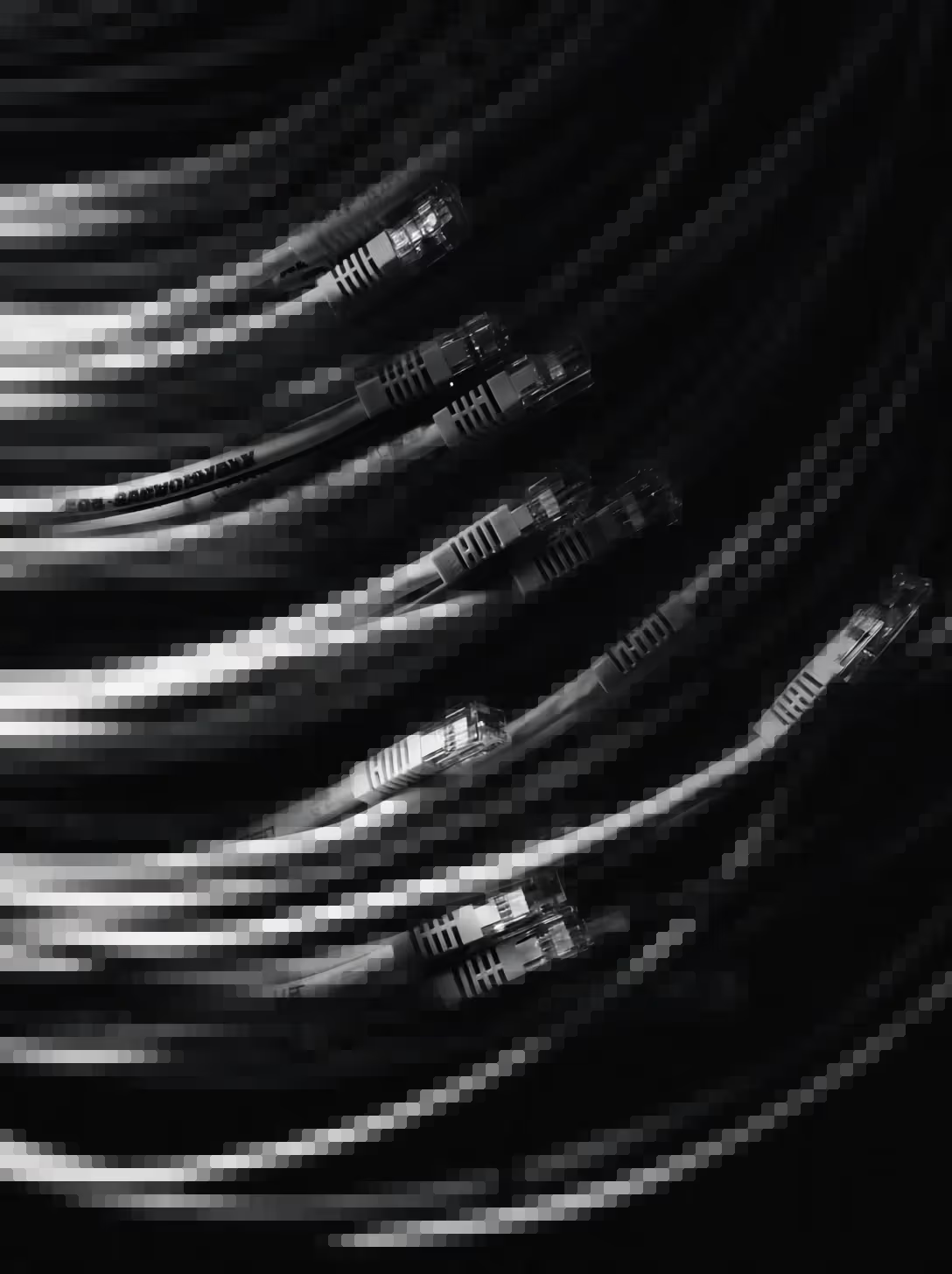
The agent-first developer toolchain: how AI will radically transform the SDLC
Nowhere has the disruption of AI been more immediate than in software development.
LLMs excel at programming because code is a structured, (mostly) deterministic language with vast training data. Unlike natural language, where ambiguity is intrinsic, software engineering follows rigid syntactic and logical rules, making it an ideal domain for AI.
Yet despite their raw capabilities, today’s LLMs haven’t drastically transformed software engineering; instead, they’ve been largely bolted on to yesterday’s tools and workflows, resulting in a shift that’s been more evolutionary in nature than revolutionary. Proverbially, it’s as if we’ve strapped a jet engine to a horse and buggy—a radical augmentation that is fundamentally limited by an outdated foundation.
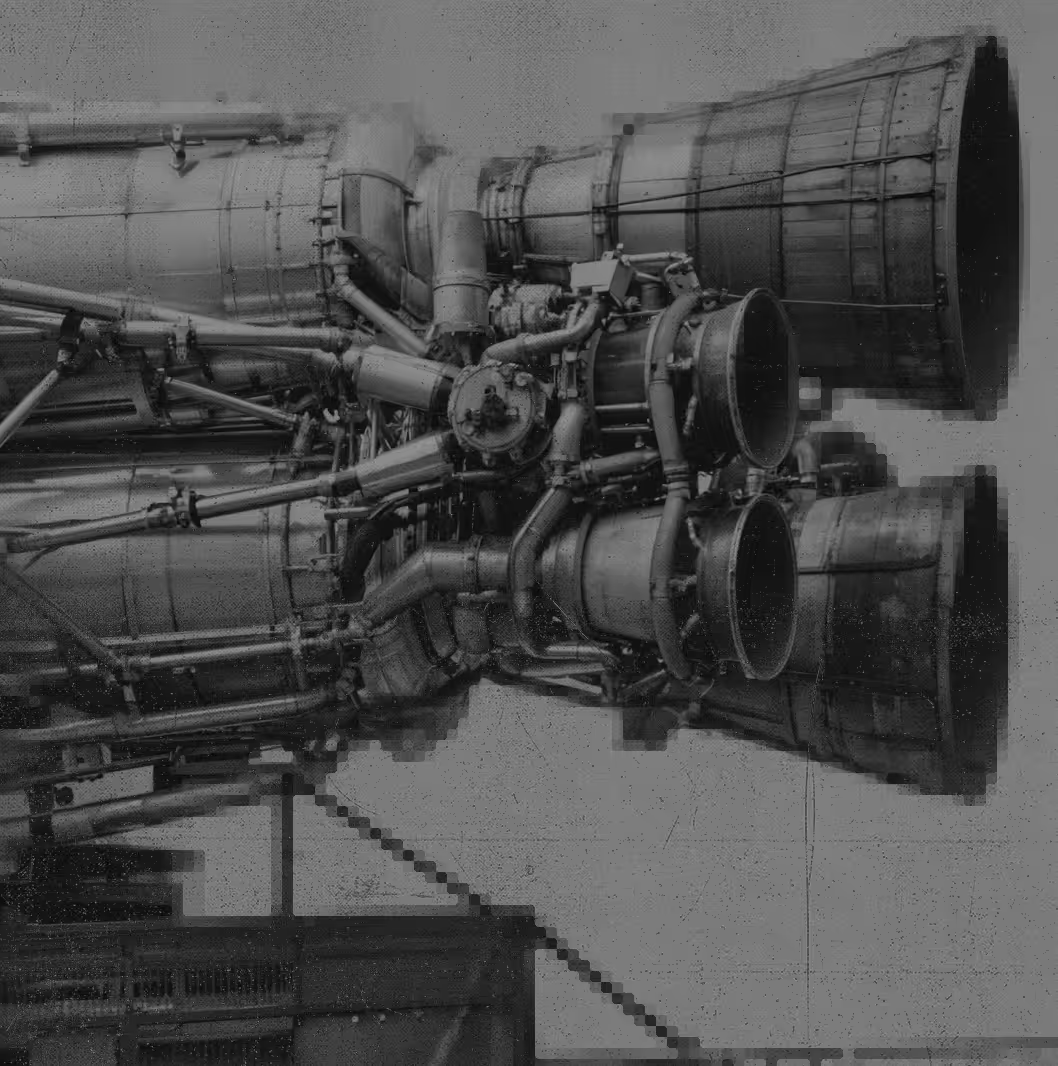
The platform shift is still very much on the horizon and will arrive once autonomous AI agents replace human developers at scale. This shift won’t be just an acceleration of existing paradigms; it will be a wholesale redefinition of how software is built.
That AI agents are going to change software engineering is not a novel take. But we’ve seen few lay out a specific vision for this future, let alone fully grasp its implications. Moving from a human-first to AI-first software development paradigm is not a mere efficiency gain; it is a fundamental reimagining of the field of software engineering. In kind, this transition will demand an entirely new developer toolchain with novel abstractions and infrastructure purpose-built for AI-first development.
The traditional SDLC—built around sequential, human-centric collaboration—is heading for obsolescence. In its place will emerge autonomous systems of specialized AI agents that continuously write, test, deploy, and optimize software at machine speed. In this paradigm, value will increasingly shift from writing code to verifying and validating code.
Critically, the core developer tools themselves—IDEs, version control systems, CI pipelines, and more—must be reimagined not as interfaces for human authorship, but as coordination layers for intelligent agents. Auditability, constraint enforcement, and real-time orchestration will become the new pillars of software engineering in an AI-first world.
It’s widely accepted that AI will upend software development. This post dives into the “how,” surfacing the fault lines most primed for rupture—and reinvention. I’ll cover:
- A (very) brief history of computer programming
- The human-centric SDLC today
- The agent-centric SDLC of tomorrow
- The future of IDEs
- The future of version control systems
- The future of CI and testing infrastructure
Let’s get into it.
A (very) brief history of computer programming
The first computers like ENIAC and the IBM 704 had no operating systems, requiring programmers to encode instructions directly in machine code—binary sequences physically punched onto cards or paper tape. Every program had to be meticulously crafted, then fed into the machine, and executed in a single batch, with no interactivity or debugging beyond trial and error.
The emergence of operating systems in the 1950s introduced symbolic programming via assembly language, offering a slight abstraction from the raw 1s and 0s of machine code. The mainframe era of the 1960s accelerated this trend, with high-level languages like Fortran, COBOL, and Lisp allowing programmers to express logic in more human-readable terms. Compilers translated these abstractions into machine instructions, and terminals gradually replaced punch cards, enabling more interactive development.
By the 1980s and 90s, programming began to resemble what we recognize today. Personal computers democratized software development, and graphical user interfaces (GUIs) made programming more accessible. Integrated Development Environments (IDEs) like Turbo Pascal and Visual Basic bundled editing, compiling, and debugging into a single workflow. Object-Oriented Programming in C++, Smalltalk, and later Java introduced modular, reusable code, while early version control systems (RCS, CVS, SVN) enabled collaboration among teams.
The Web and cloud era transformed development again. JavaScript, PHP, and Python fueled web applications, open-source software proliferated, and Git and then GitHub revolutionized developer collaboration with distributed version control. Cloud platforms like AWS abstracted infrastructure, enabling on-demand, always-available, scalable compute and storage. DevOps then merged development and operations, with CI/CD pipelines automating testing and deployment, and Infrastructure as Code tools like Terraform making infrastructure programmable too.
The trend over the last half century has been towards ever-higher abstractions, to make the creation of code simpler. Yet through every evolution, one principle has remained constant: software development was for humans. Every tool—new languages, compilers, IDEs, version control, etc.—was created to make human programmers more effective. More so, the very notion of software engineering has always been tied to writing code. But as AI increasingly demonstrates the ability to generate, debug, and even architect software, this foundational assumption is beginning to unravel.
The software development lifecycle today
The software development lifecycle (SDLC) takes many forms, but most modern workflows follow a familiar loop:
- Write Code – Developers start by cloning a central repository from their version control system (e.g., GitHub, GitLab) and creating a new branch (git checkout -b feature-branch) to isolate their changes. They write code in an IDE (e.g., VS Code, JetBrains) with local linters and unit tests helping to catch errors before committing.
- Test Code – Developers commit (git commit -m "Add feature") and push (git push origin feature-branch) their changes to the remote repository. This triggers Continuous Integration (CI) pipelines (GitHub Actions, Jenkins, BuildKite) that run unit tests, integration tests, static analysis, and security scans.
- Review Code – A Pull Request (PR) is opened, initiating peer review. Team members review the changes, provide comments, and request modifications.
- Merge Code – Once approved, the PR is merged into the main branch (git merge feature-branch).
- Deploy Code – Merging to main triggers Continuous Deployment (CD) pipelines, which package and deploy the software to staging or production, generally in a cloud environment like EC2, Heroku (RIP), Kubernetes, or Lambda
- Monitor Code – Once deployed, observability tools (Datadog, Prometheus, Splunk) track performance, errors, and logs. Alerts notify engineers of incidents, and insights from monitoring feed back into new development cycles.
- Iterate – Based on feedback, new issues are created, branches are spun up, and the cycle repeats.
Every stage of this pipeline is built around a sequential, human-centric model: teams of engineers collaborating on shared codebases, reviewing each other’s changes, and iterating in well-defined cycles. But in a world where AI agents generate, modify, and deploy software autonomously—at speeds and scales far beyond human capability—much, if not all, of this workflow becomes obsolete.
The question is: what does software development—and the SDLC specifically—look like when machines, not humans, are writing the code?
The software development lifecycle tomorrow
It's hard to make predictions, especially about the future.
- Yogi Berra
While predicting the exact contours of an AI-first SDLC is an exercise in pure speculation, certain foundational shifts are inevitable and we can make bolder claims about those.
For starters, the transition from human-led development to the agent-driven paradigm will be defined by real-time collaboration between specialized AI agents, continuous self-correction loops, and an unprecedented level of automation. Software development will no longer be a sequential pipeline of rigid steps aiming to synchronize human effort but rather an autonomous, round-the-clock system that continuously generates, tests, deploys, and optimizes code…with human guidance and supervision, of course.
Rather than developers manually writing code, submitting pull requests, waiting for CI/CD pipelines to execute, and addressing human review comments, swarms of agents will work in unison—building, reviewing, and integrating changes in real time. These agents won’t merely generate code but will also validate their own work by running extensive test suites, benchmarking performance, and refactoring as needed.
The human role in software development, meanwhile, will shift from writing and reviewing code to defining intent, constraints, and policies and then validating the work of machines. Engineers will spend more time specifying system behavior in natural language and/or formal requirements, while AI agents translate these directives into executable software.
In this paradigm, value will increasingly shift from writing code to verifying and validating code.
Realizing this future necessitates a substantial evolution in software infrastructure and tooling. We anticipate the emergence of entirely new categories of developer platforms, sophisticated agent-orchestration frameworks, specialized observability systems for autonomous workflows, and automated mechanisms for conflict resolution. From IDEs and version control systems to programming languages, runtimes, and CI/CD pipelines, these foundational elements will undergo transformative change—rendering many of tomorrow’s tools unrecognizable compared to today’s counterparts.
Let’s dive into some specific areas we foresee are ripe for massive change, particularly looking at the IDE, VCS, and CI and testing infrastructure.
IDEs
The IDE is where the modern developer lives; it’s where work gets done. IDEs have become all-in-one hubs that streamline coding, often combining a source code editor, build automation, and debugger into one application.
More recently, IDEs have begun incorporating AI as a coding copilot. For example, GitHub Copilot integrates into editors like VS Code and IntelliJ to offer auto-completions and even generate entire functions based on comments or context. It acts as an “AI pair programmer,” suggesting code, tests, or comments as you work
Tools like Cursor and Windsurf—built from the ground up to enable a more interactive, AI-driven coding experience—enable inline AI modifications, allowing developers to rewrite, refactor, or debug sections of code dynamically with the help of AI.
However, these AI-powered features still serve the human developer—accelerating rote tasks like writing boilerplate code, generating unit tests, refactoring code or documenting code—while keeping the developer in control. Even Cursor’s “agent mode” is not really a full fledged agent; it’s still human driven development. In essence, today’s IDE is a human-centric cockpit with emerging AI aids on board.
Agent-first IDEs will fundamentally differ by transforming software development from explicit, syntax-driven coding into high-level intent specification, conversational interaction, and proactive AI-driven automation.
You can envision the IDE of the future will be:
- Conversational: Code emerges from ongoing dialogues, not just static files.
- Intent-oriented: Focus on developer’s intent and outcomes rather than syntax.
- Multi-player and orchestrative: IDEs manage multiple specialized AI agents that perform discrete tasks (e.g., architecture, testing, security, optimization).
- Interactive and reactive: Agents proactively propose and execute changes rather than waiting for explicit commands.
What might this IDE of the future look like and what will be key features? A few things I expect to see:
Features for an agent-first IDE
- An intent specification layer. Agents don't just need prompts—they need structured, machine-readable intent representations. The IDE must support DSLs for describing desired outcomes, tools to define constraints like budget limits and performance SLAs, and the ability to compare current system state to desired goals, and let agents plan accordingly.
- Agent-framework integrations. Instead of plugins for tools like linting and debugging, future IDEs will integrate with agent frameworks—systems like Cody, Devin, Claude Code, or custom LLM pipelines. These integrations will support UIs for configuring agent roles and permissions, interfaces for visualizing and steering how different agents (e.g. frontend, backend, infra) are collaborating, and persistent agent memory that allows long-term project context across sessions.
- Agent monitoring and telemetry. Agents need constant monitoring to ensure correctness, safety, and alignment. We will need live dashboards to visualize agent tasks and status, trace-based debugging to step through an agent’s reasoning chain and code generation path, and alerts on unexpected behaviors like unsafe code or violating performance bounds.
- Explainability and decision logging. In a world of autonomous agents, trust depends on transparency and fine-grained auditability; more on this below. Agents will need to output action logs for each input, output, and rationale. We will want interactive code diff explanations (“why did this code change?”), plus some basic system summaries: the ability for agents to overview architecture, data flows, etc.
- Automated testing & verification. This is the subject for an entire other blog post, but the IDE of the future needs to be able to verify agents. This verification needs to cover code tests (unit, integration, etc.), and support simulated sandbox environments to validate code behavior before merging. And finally, IDEs will need to support formal verification methods like TLA+ and ROCQ.
- Live collaboration and feedback loops. For humans and agents to work well together, we’ll need real-time synchronization via real-time agent-human chat (like Copilot chat, but system wide), editable simulations that let you preview a feature and leave feedback (like in Google Docs or Figma), and live agent tuning via adjusting hyperparameters, toolsets, or constraints.
- Knowledge graph and memory integration. Agents will need structured knowledge about the system, business logic, and users. I expect to see things like a semantic project graph, where the codebase (functions, models, services) is represented as an interconnected knowledge graph. We will also need embedded documentation plus persistent long-term memory that allows agents to remember past decisions, tradeoffs, and user preferences.
- A pluggable execution environment. The IDE of the future needs a sandbox where agents can safely run and test code. Examples include containerized runtime environments, policy enforcement engines to restrict what agents can deploy or access, and a tooling-calling interface layer that lets agents use CLI tools, APIs, and libraries with traceable logs.
Think of this new IDE as a mission control center for intelligent systems; the developer becomes a strategist, curator, and verifier rather than a code-writer.
Version Control Systems
The version control system (VCS) is the heart and lungs of modern software development; it’s where code hosting, collaboration, history tracking, and continuous integration converge. Systems like Git enable developers to coordinate changes, revert errors, and merge divergent code paths seamlessly.
But the version control platforms we use today, and the enabling workflows around them, are built explicitly around human-driven interaction, collaboration, and decision-making. It becomes clear that these tools and workflows begin to break down as we shift to an agent-first paradigm of software development.
First, agents generate vastly more commits than humans, quickly overwhelming the pull-request-oriented workflow designed for manual review. The sheer volume of changes becomes impractical to track manually. Branches will proliferate uncontrollably, increasing merge complexity and decreasing the readability of commit histories.
Further, traditional Git workflows rely heavily on human reviewers assessing code quality and correctness through pull requests. With AI agents continuously producing code, manual code reviews become a bottleneck.
Finally, traditional VCS treats merges as line-by-line textual merges, not semantic merges. AI-generated code often requires understanding code intent, logic flows, and system-wide interactions, beyond textual diff tools. Frequent merge conflicts arise, demanding nuanced resolution that traditional text-based merge conflict tools cannot handle effectively, causing workflow disruptions.
Net net, today’s VCSs are simply not equipped to handle the scale, velocity, concurrency and complexity of the agent-driven model. This all begs the question, what does the next generation Git resemble?
Features for agent-first version control
The future VCS will need to be intent-aware, conflict-resilient, and built for autonomous negotiation and coordination. Here are some defining characteristics:
- Everything is a commit. Instead of the canonical atomic unit being a file, tracking needs to shift to be action centric. Every agent action gets committed in a structured ledger, which improves traceability, auditability and helps adapt to the high frequency operations we’ll see from agents.
- Autonomous, ephemeral branching. Agents will need the ability to create their own branches for individual tasks. These branches will exist only as long as needed for task completion, and may be intertwined with continuous “local” branching that each agent uses to maintain a local thread integrated into a central system.
- Semantic merging > textual diffing. An agent-first VCS needs changes to be based not on file edits, but instead on the change’s effect on a system’s behavior and goals. Conflict resolution between branches needs to happen at the architecture, API, or system level, not the file level. For example, imagine two agents change the same functions, but one improves latency while the other improves readability—the system can test both and decide based on specified SLAs.
- Constraint-driven conflict resolution. This is related to semantic merging. Instead of blocking on a merge conflict, the system should evaluate whether multiple changes can coexist under global constraints like performance or cost. If not, agents can “negotiate” alternatives or escalate to humans.
- CI by default. Like the ideal we’ve all been chasing for years, in an agent-first VCS, every commit is validated in a simulated runtime environment. If it passes tests, constraints, and trust thresholds, it’s merged automatically; the idea of a pending PR disappears, as the system is always in flux and validating in real time. More on this in a bit.
- Intent and rationale versioning. In the same vein as semantic merging, commits are no longer diffs: they’re intent snapshots, capturing what the agent was trying to achieve. This allows the system to trace regressions to decisions, not just contextless file changes. It also lets you rewind the software based on goals (e.g. “undo the optimization that introduced latency in v14”).
- Collaboration via shared memory, not PRs. Agents and humans will collaborate through a shared semantic model of the overall system. Instead of PRs, agents might subscribe to events, watch for constraint violations, or join planning threads that are related to goals.
- Agent identity, trust, and auditing. Authentication today is based on who has repository access. In an agent-first world, every change will need to be signed by the agent who authored it, with extensive metadata: agent version and configuration, a confidence score, and evaluation results. Different agents might develop trust scores over time, which would influence whether their changes are accepted automatically or require escalation.
Whereas today Git is a versioned text store, future systems will be:
- A distributed intent-resolution engine
- A constraint-aware, trust-governed merge system
- A collaborative memory layer for human-agent software evolution
These systems won’t just track what changed, but why, who initiated it, what constraints it satisfied or violated, and how it affects the system holistically.
CI & Testing
Continuous Integration (CI) and testing pipelines serve as the guardians of quality in today’s SDLC. They provide critical feedback loops that catch regressions, enforce coding standards, and verify system correctness and security before changes are merged and deployed. Tools like GitHub Actions, Jenkins and BuildKite automate the execution of unit tests, integration suites, static analysis, and security scans—ensuring human-authored code meets baseline quality gates.
But these pipelines were built for a world where human engineers write code at a predictable pace and where pull requests and manual reviews serve as the primary decision checkpoints. In a world where AI agents are autonomously generating thousands of changes per hour, running in parallel across system boundaries, the traditional CI model doesn’t work.
CI in an agent-first world is not a periodic pipeline—it’s a continuous, real-time validation layer. Every agent action must be verified immediately and automatically, evaluated not only against functional correctness but also system-wide constraints like latency, cost, risk exposure, and business logic compliance.
As such, testing and verification cease to be a gate at the end of a workflow and instead become a fabric woven throughout the system—an always-on validation system that enables autonomous iteration with confidence.
Let’s explore what an agent-first CI & testing infrastructure might look like.
Features for agent-first CI & testing infrastructure
- Simulation-first testing environments. Agent-generated changes need to be verified in ephemeral, sandboxed runtime environments—essentially high-fidelity digital twins of the production system. These simulation layers enable testing code behavior, dependency interactions, and performance characteristics safely, before deployment. In many cases, rather than just sandboxing code, the runtime needs to be fully deterministic to support exhaustive search. This allows agents (or humans) to reliably reproduce failures and iterate safely.
- Always-on, agent-level verification. Each agent should be embedded with its own local CI loop, validating its work continuously before contributing to the shared system. Instead of waiting for a push to the main branch, verification happens in real time, on the change level: every proposed change is simulated, benchmarked, tested, and compared against historical baselines and stated goals.
- End-to-end property testing and auto-spec synthesis. In a world where human-written specs are rare or outdated, CI systems must infer specifications from existing behavior, logs, and documentation. Agents should automatically generate property-based tests, fuzz inputs, and validate edge cases at scale based on the specs they were created from.
- Deterministic Replay and State-Space Exploration. A critical new feature is the integration of deterministic replay and state-space exploration tools. In large-scale autonomous environments, traditional CI struggles with flaky tests and elusive bugs. By incorporating tools that offer deterministic replay, we’ll create highly controlled runtime environments where every change can be reproduced exactly. This allows the CI system to:
- Replay Execution Paths: Reconstruct the precise sequence of events leading to a failure, ensuring that bugs are not only reproducible but also isolated to their test cases.
- Explore the Full State Space: Utilize state-space exploration to automatically generate edge cases and stress tests, uncovering failure modes that wouldn’t surface in standard testing.
This deterministic layer serves as an automated adversarial testing subsystem. It methodically explores every available execution path, ensuring that the software behaves reliably under all possible conditions—a capability essential when agents are iterating at machine speed. Tools like Antithesis (and deterministic simulation testing in general) become central to the entire software development process.
- Integrated formal verification, especially for critical paths. For critical workflows—auth, payments, safety loops—formal methods will gain prominence. Agent-first CI pipelines will support Lean, TLA+, Rocq, or new agent-friendly DSLs that allow verification of invariants, state transitions, and safety guarantees. This formal tooling will be deeply integrated into the CI system—not a niche tool used by specialists.
- Constraint-based validation, not just test pass/fail. A single green test suite is insufficient when agents are optimizing across competing goals. Validation needs to incorporate business constraints (e.g., "keep infra cost under $5k/mo"), performance SLAs, and compliance policies. CI becomes a multi-objective decision engine—ranking and filtering changes based on a combination of test results, optimization targets, and system constraints.
- Trust-based merge gating. As discussed in the VCS section, you can envision that different agents will accumulate trust scores over time. The CI system will integrate these scores to decide which changes can be merged automatically, which require shadow deployment and monitoring, and which should be escalated to humans for review. CI becomes not just a validation tool but a dynamic policy enforcer.
- Live testing and rollback-aware deployment. CI doesn’t end at merge. As code moves into production, live traffic testing, canary releases, and automatic rollback mechanisms become essential. CI pipelines will integrate observability hooks and real-time monitors to detect anomalies, triggering automated rollbacks or retraining of misaligned agents.
Whereas today’s CI tools check whether the code compiles and passes tests, tomorrow’s systems will ask deeper questions:
Can we trust it to run without intervention? Does this behavior align with intent? Does it improve the system holistically?
Where software development is going
The shift to agent-led software development is not a faster horse—it’s a new mode of transportation entirely. In this future, the core artifacts of the SDLC—IDEs, version control systems, CI pipelines, etc.—don’t just get smarter. They get reimagined as coordination layers for intelligent agents, not interfaces requiring human input.
The IDE becomes a mission control hub for orchestrating autonomous collaborators. The version control system transforms into a semantic ledger of agent intent and negotiation. CI evolves into a continuous, constraint-aware trust engine—backstopped by deterministic simulation and formal guarantees.
The developer’s role doesn’t disappear—it ascends. From code author to system architect. From debugger to intent designer. From manual reviewer to the human-in-the-loop of a complex, intelligent system.
The future of software development isn’t built around code—it’s built around real-time coordination.