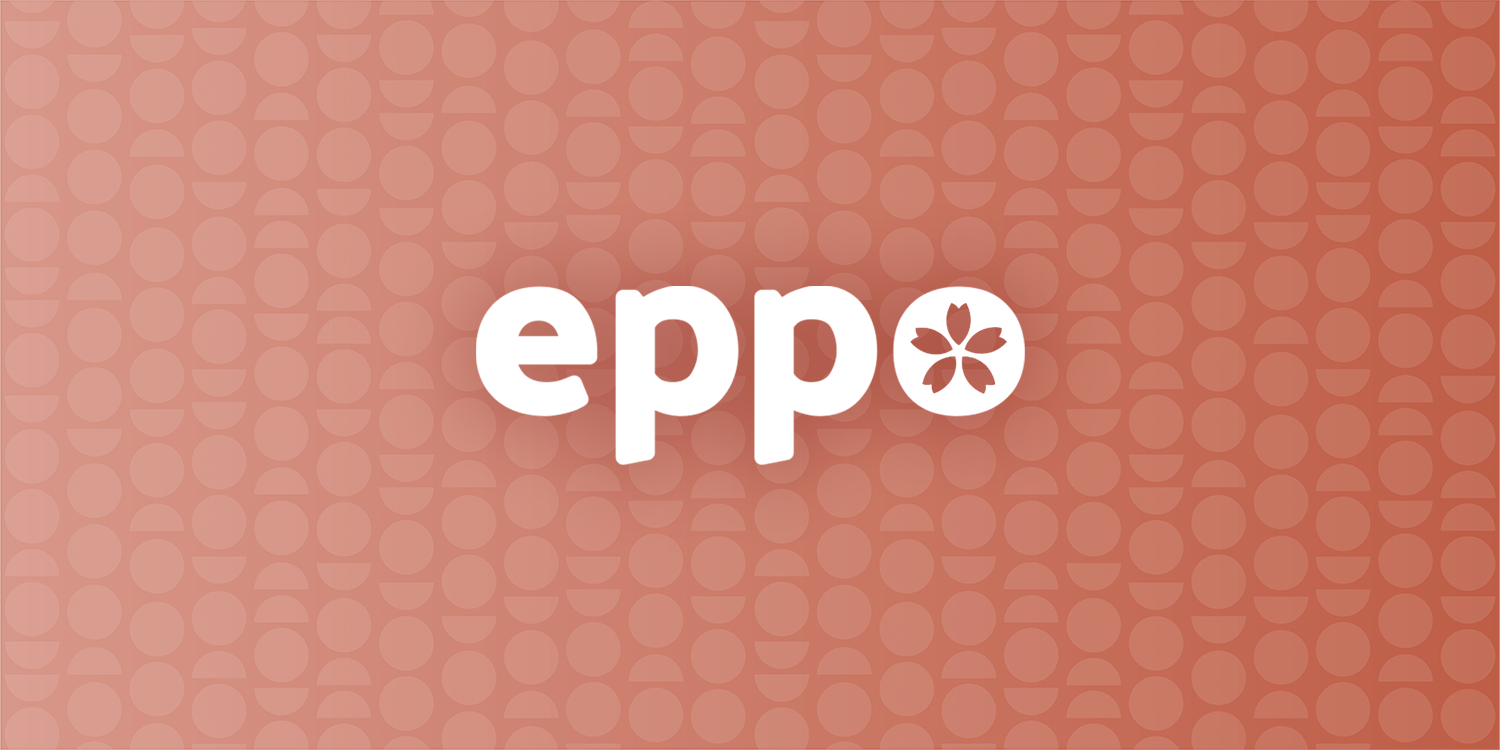
Our Investment in Eppo
Some of the most successful web-scale companies of the past decade have developed advanced experimentation platforms in-house to make better decisions with data (see Google, Netflix, Airbnb, Uber, Twitter, Spotify, Pinterest, DoorDash). Experimentation may be the clearest way for data science teams to drive bottom-line impact (i.e., revenue). Why do so many companies build their own experimentation stacks instead of leveraging an off-the-shelf solution?
We spoke with data and experimentation teams across startups and larger companies and found that there are (at least generally) six major components of a modern experimentation stack:
- Telemetry – instrumentation that provides a systematic way to track and collect events.
- Feature flagging – a method to remotely configure features on and off and control who sees what.
- Assignment service – responsible for bucketing users into different variants and properly randomizing an experiment’s traffic to ensure results are statistically unbiased.
- Stats engine – an engine that powers and enforces statistical analysis such as which tests to use, significance, etc.
- Metrics repo – a metrics repository to define and keep track of commonly used metrics. This includes guardrail metrics and product-specific metrics. Some systems also allow on-the-fly metrics calculation.
- Analysis UI – a visual interface to view, explore, and control experiments. This includes a way to analyze the results of experiments on different metrics and, in some cases, the ability to ramp up and down traffic.
In 2020, after validating that experimentation was a revenue driver for many web-scale companies with strong executive support, our investment team set out to research the systems that existed for high-growth companies to support experimentation cultures. We found that the off-the-shelf options lacked analytical rigor for deep product experimentation or were overly complex to integrate. A few other interesting things we found:
- Heterogeneity is overestimated: Companies that build experimentation platforms often adopt a similar design based on six key components outlined above (telemetry, feature flagging, assignment service, stats engine, metrics repo, analysis UI).
- Modular problems, modular solutions: Some of these components, like feature flagging, can power other use cases (like safe feature rollouts). As such, companies are reluctant to adopt end-to-end solutions that don’t integrate with their existing data stack and would prefer to adopt modular solutions that are optimized for the layers they don’t have.
- Experimentation differs between B2B and B2C: Experimentation is becoming increasingly critical for B2B companies that go bottoms-up (and B2C companies with less user traffic) as they have fewer touchpoints with their customers. These companies have unique needs related to the statistical engine and analysis UI.
- No suitable commercial or OSS experimentation solutions exist for many teams: Most teams would prefer not to build in-house, but existing tools don’t integrate easily with their data stacks (e.g. feature flagging tools, data warehouse) or meet their analytical needs (e.g. automated diagnostics, outlier detection, self-serve investigations).
Along the way, we met Chetan Sharma. Che spent his career building experimentation tooling at B2C and B2B companies like Airbnb and Webflow, experiencing firsthand the impact that data-driven culture has on product development. When we met, he was developing a vision around making experimentation accessible to any company – so that any person could test new product ideas (after all, Eppo stands for “Every Paid Person’s Opinion”). Among his previous work, he created the widely popular Airbnb Knowledge Repo. He’s committed not only to helping companies run experiments to make product decisions but also to helping teams build and access institutional knowledge gleaned from experiments. In many ways, Che is an archetypical Amplify founder — he encountered a problem, designed a solution, and wanted to make that solution accessible to the rest of the world.
Over the course of several months, we got to know Che more and were particularly drawn to his approach targeting two largely unaddressed areas: the statistical engine and analysis UI. From our prior research in this space, we believed this was the most unsolved part of the experimentation stack and required someone with deep statistical know-how to execute. When Che vocalized that he was ready to start a company, we jumped at the opportunity to partner with him.
Che started Eppo in late 2020 and quickly assembled a team of all-stars with experience at companies like Strava, Slack, and Snowflake. Historically, top-notch, high-frequency experimentation was only reserved for companies who could afford to build systems in-house and staff an internal team to support the infrastructure. Eppo flips this narrative. The Eppo team designed an experimentation platform that allows companies to connect directly to their data warehouse and leverage a statistical engine and analytics UI to handle advanced use cases (e.g. guardrail metrics, automate power analysis, CUPED). With Eppo you get:
- A dashboard to track, filter, and manage all running experiments
- An SDK to randomly assign subjects to your experiment variations
- A page for each experiment where you can track progress, metrics, and traffic allocation
- An explore panel for diving into experiment metrics and splitting across dimensions
- A metrics section for tracking and managing all experiment metrics with historical context
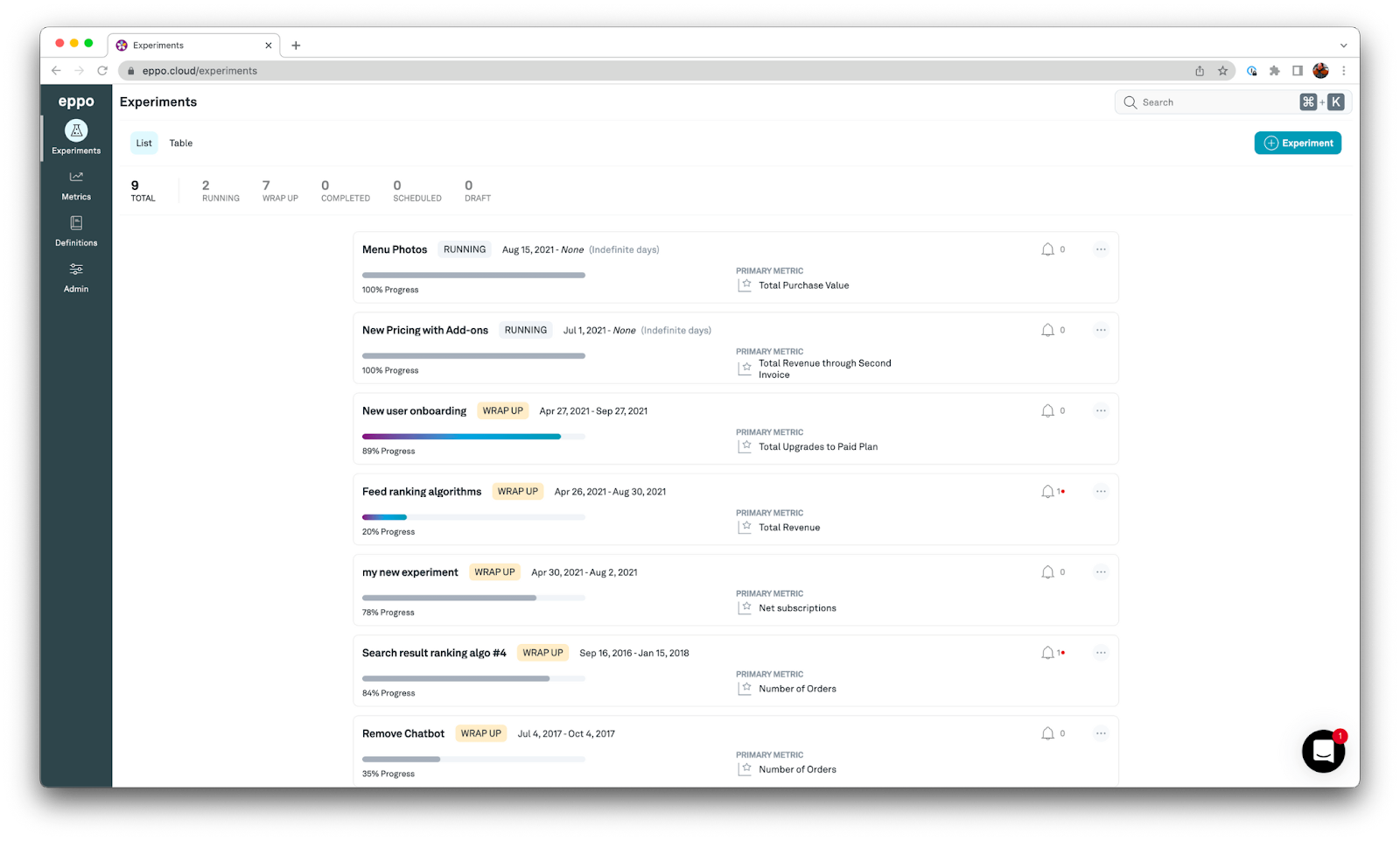
Since its founding, they have brought on a wide range of customers with different data volumes and use cases including Cameo, Foxtrot, and Netlify. Eppo is the tool that every company should use in their experimentation stack – which is why we’re thrilled to announce our seed and Series A investment in the company!

Our Investment in OctoML
We invested in OctoML because they radically change this dynamic by providing a universally accessible bridge between AI software and hardware.
By Sarah Catanzaro
April 3, 2020
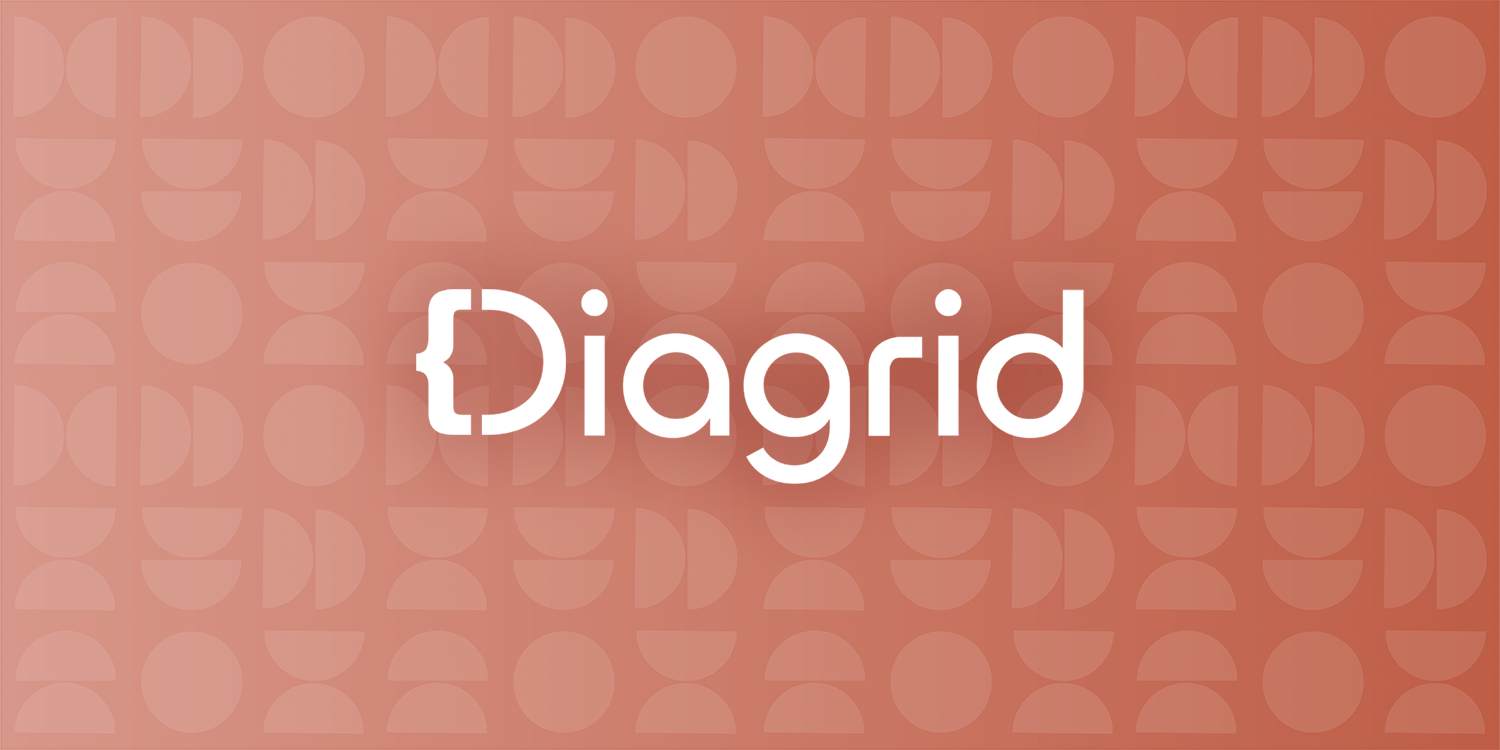
Our Investment in Diagrid
With Diagrid, any container or serverless runtime becomes a full-fledged application platform; you can kiss these Frankenstein architectures “goodbye!”
By Lenny Pruss and Renee Shah
October 12, 2022
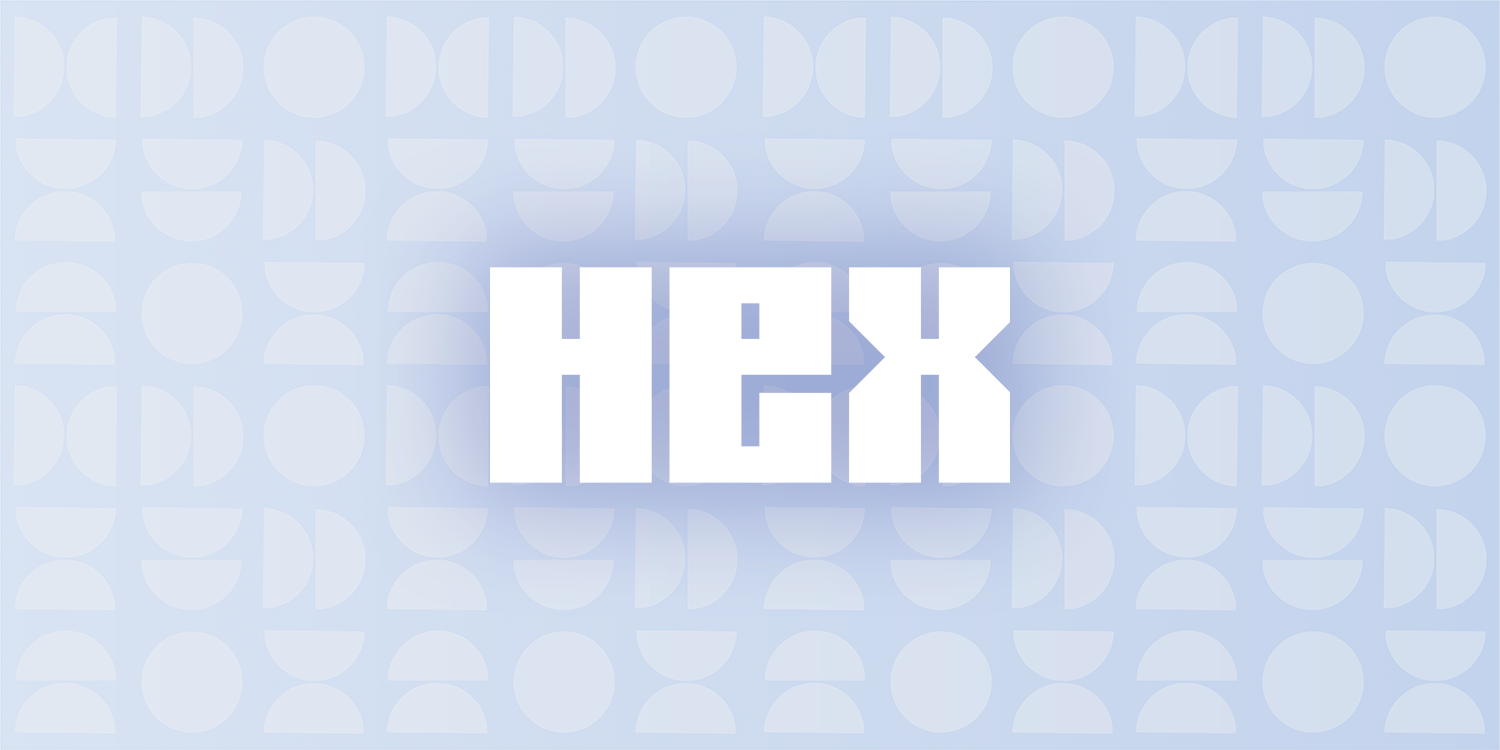
Announcing Our Investment in Hex
Hex helps data teams drive impact by improving productivity, facilitating collaboration, and making it easy to create beautiful, interactive data apps and stories.
By Sarah Catanzaro
March 31, 2021